Christina Sewall
GIS Portfolio
Remote Sensing: Image Analysis and Classification
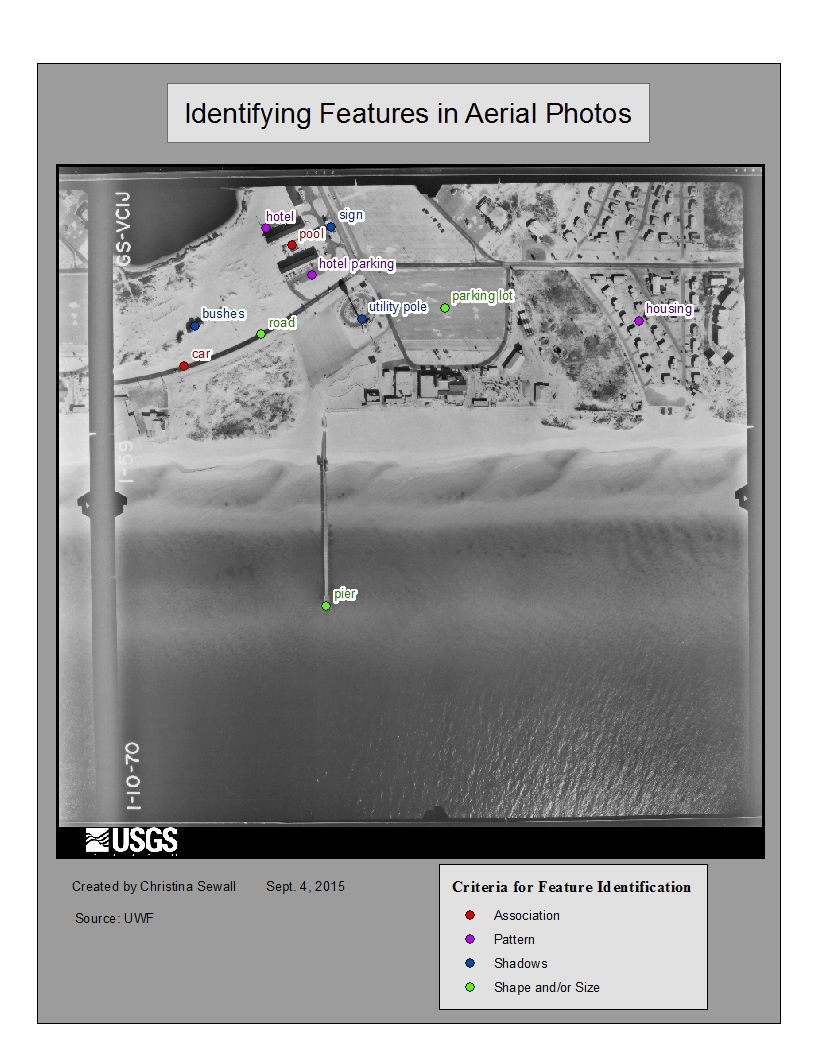
Identifying features in aerial photos using shape and size, shadows, patterns, and association
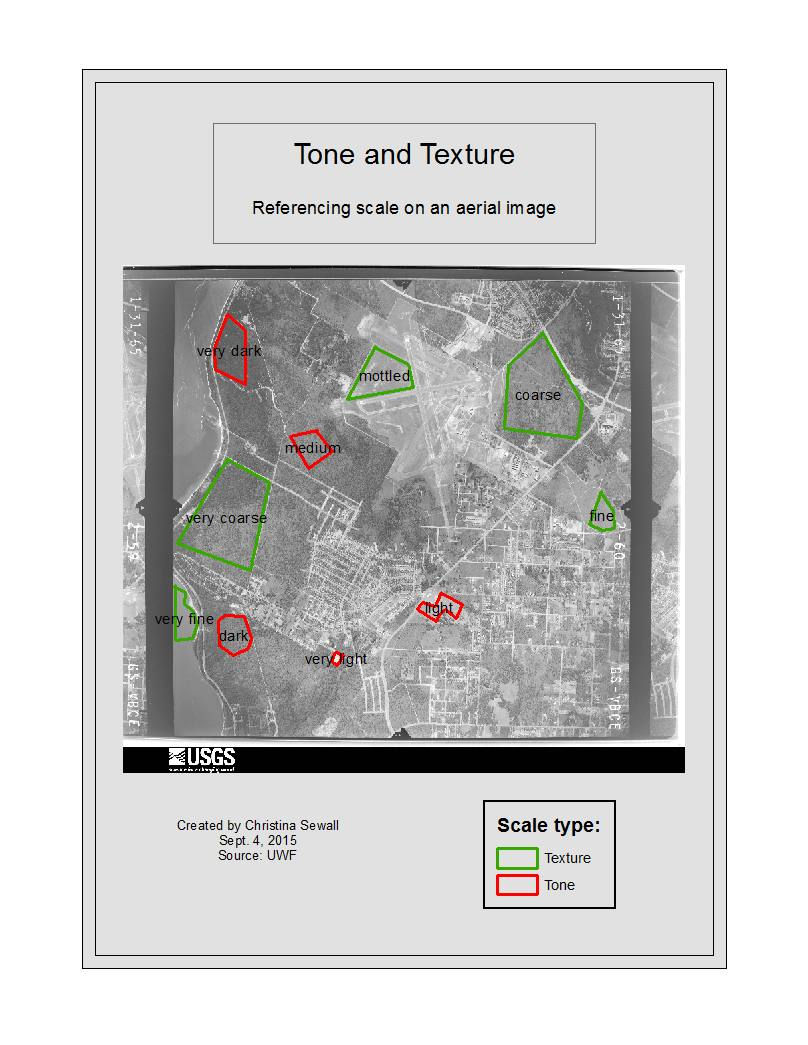
Creating of two scales, one for tone and one for texture, based on interpretation of an aerial image
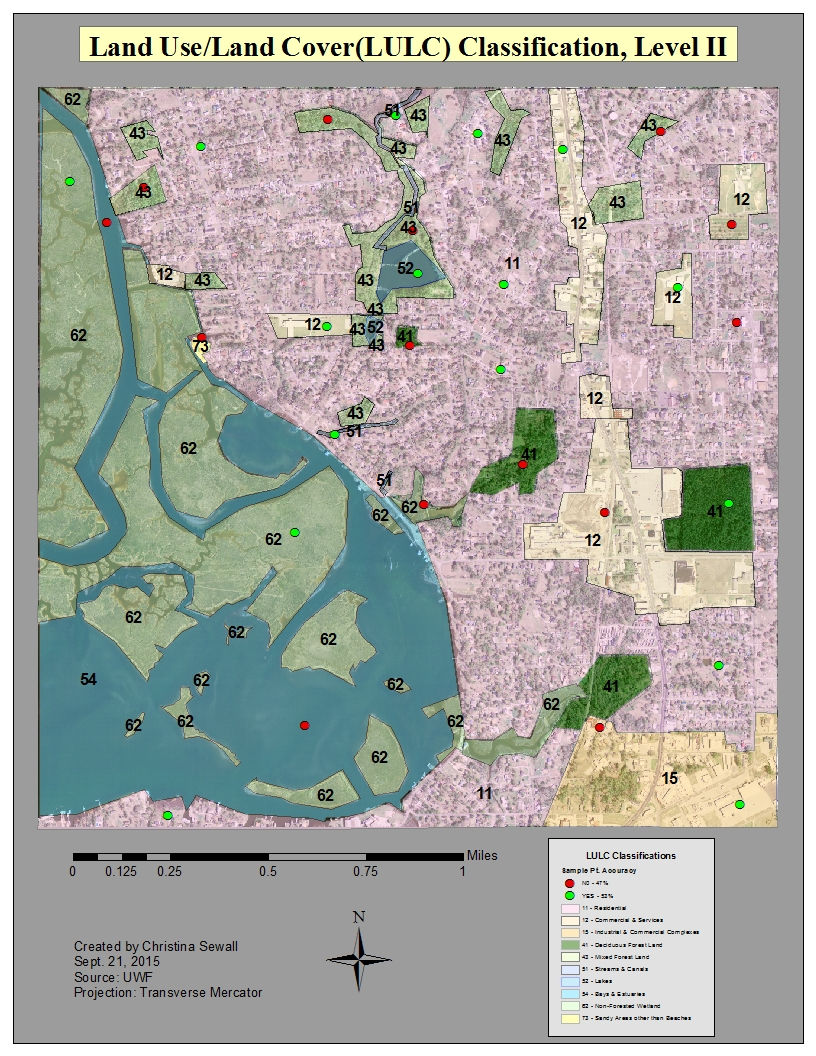
Land Use/Land Cover Classification based on identification of features using tone, pattern, shape, etc. from which LU/LC classes were made. The accuracy of this classification was checked using Street View in Google Maps.
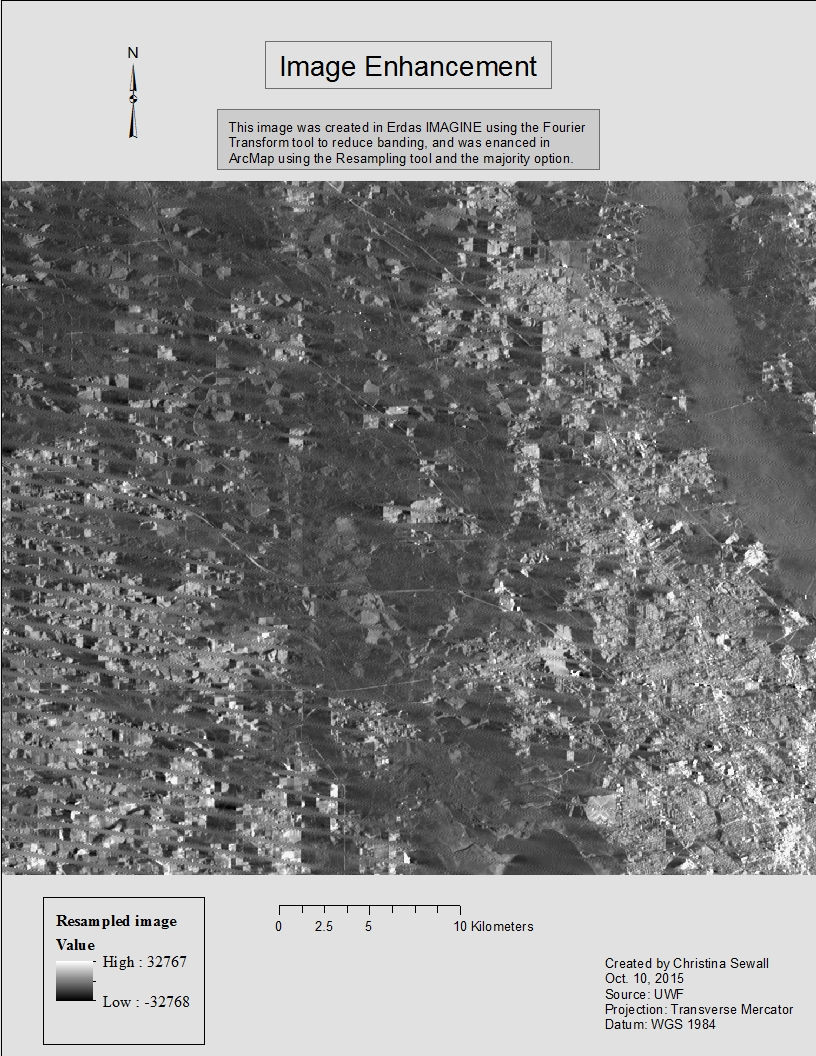
Map of a Landsat7 Image enhanced using ERDAS Imagine and ArcGIS to remove striping and increase contrast and detail.
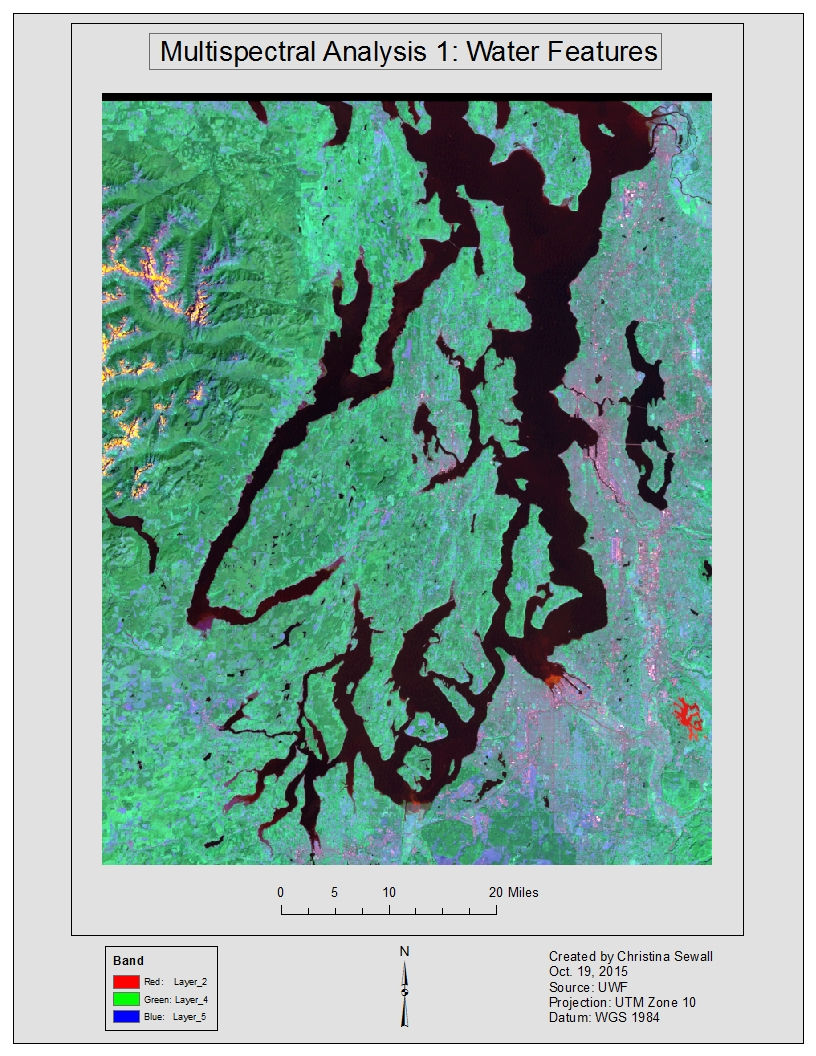
Histograms were used to identify spectral characteristics of water features. Bands 2,4, and 5 highlighted these features.
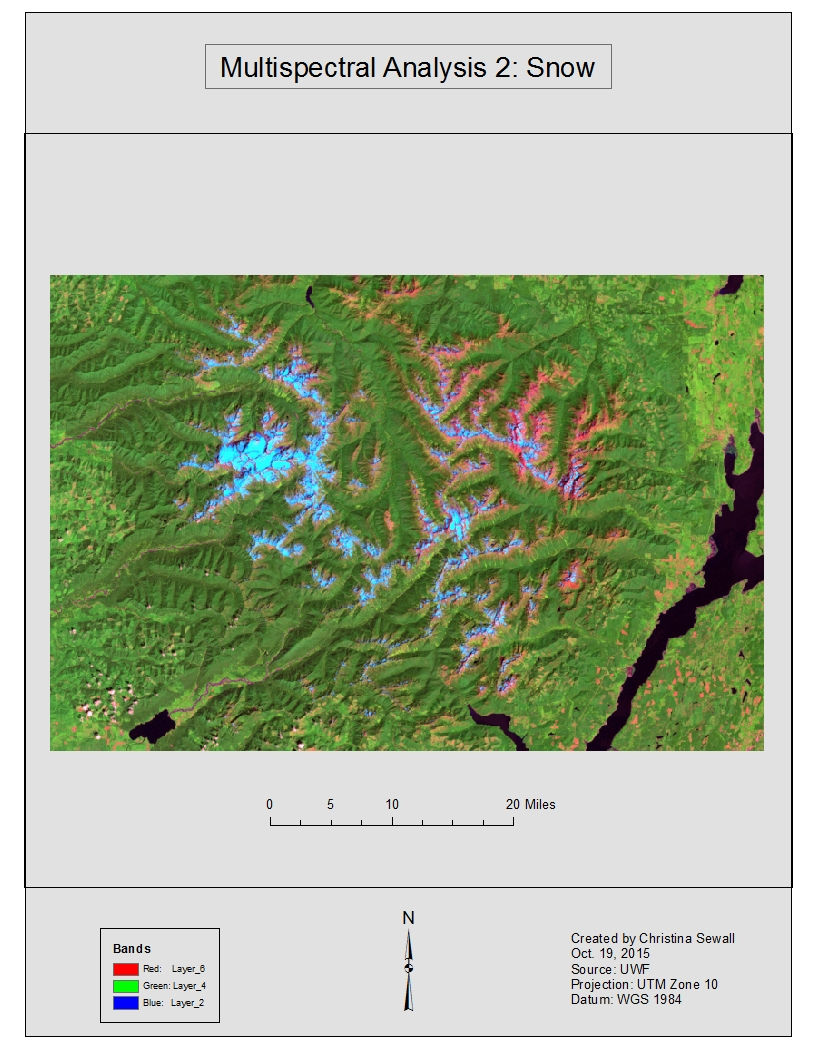
The snow is highly reflective, highlighted by Bands 6,4, and 2.
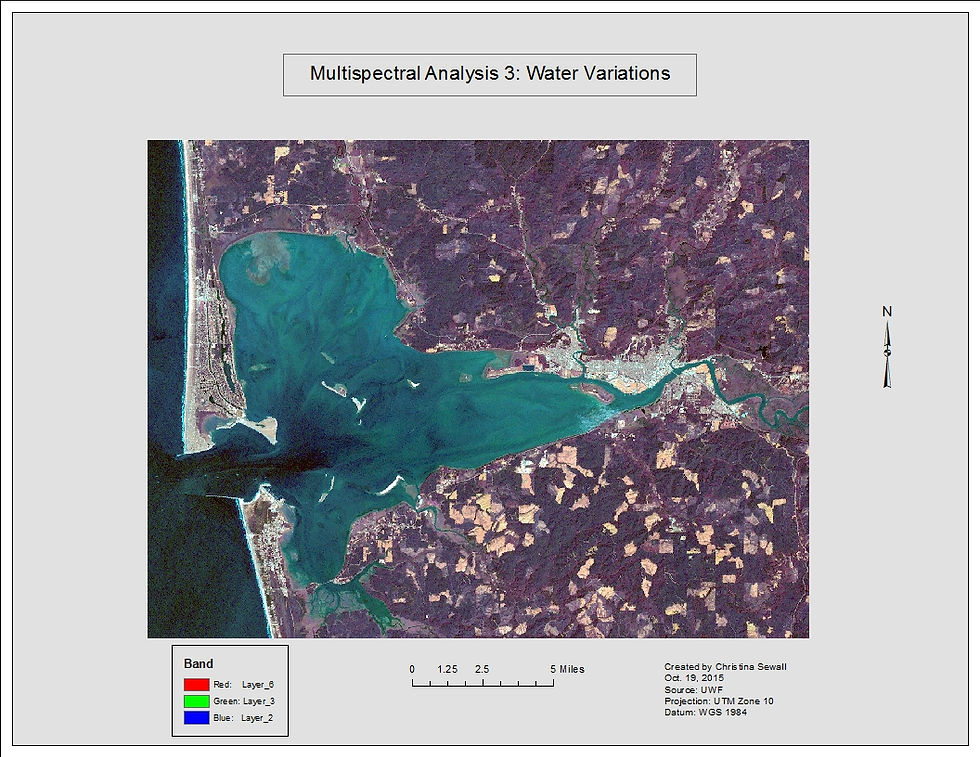
Bands 6,3,2 in combination show the variations within water features; vegetation stays muted.

One image is highlighted 3 ways to examine different areas of interest.
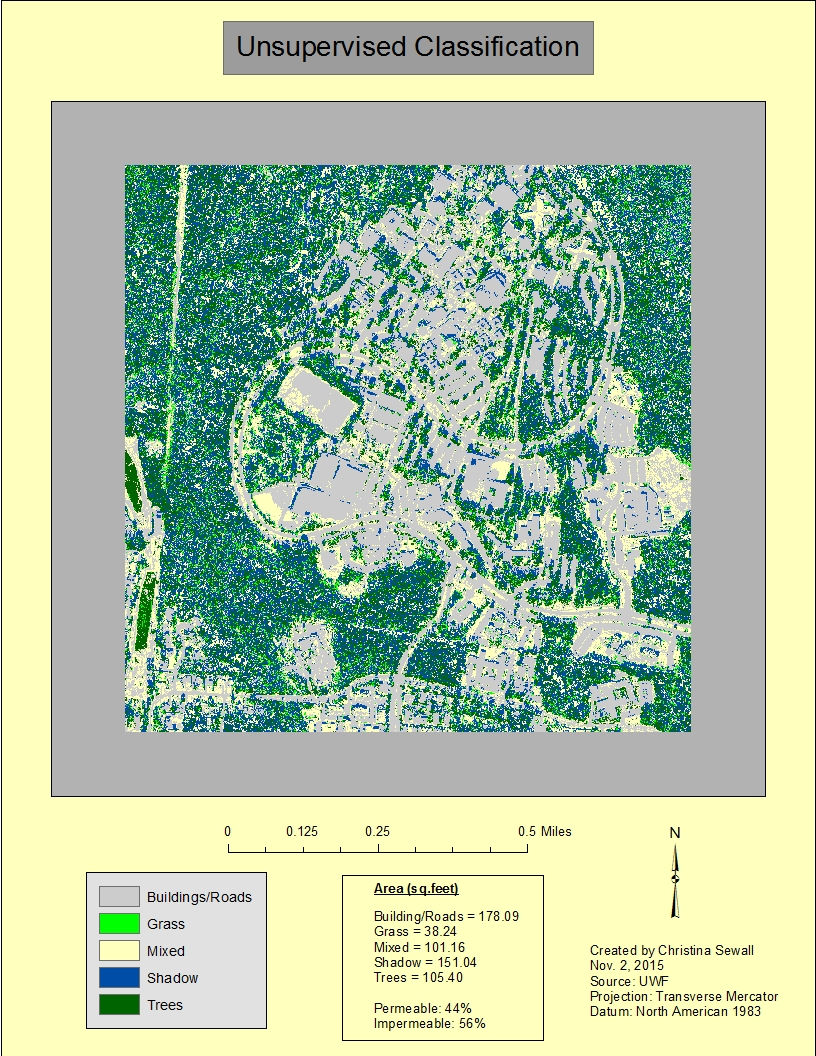
Here a Landsat image was processed so that clusters of similar pixels were grouped as classes, and then those classes were matched to various feature types in the original image. The result is a thematic map representing, in this case, 5 classes. The classes can be analyzed to answer questions; in this case the question was what percentage of the image represented impermeable versus permeable surface. The necessary information was contained in the Attribute table for the newly created classif
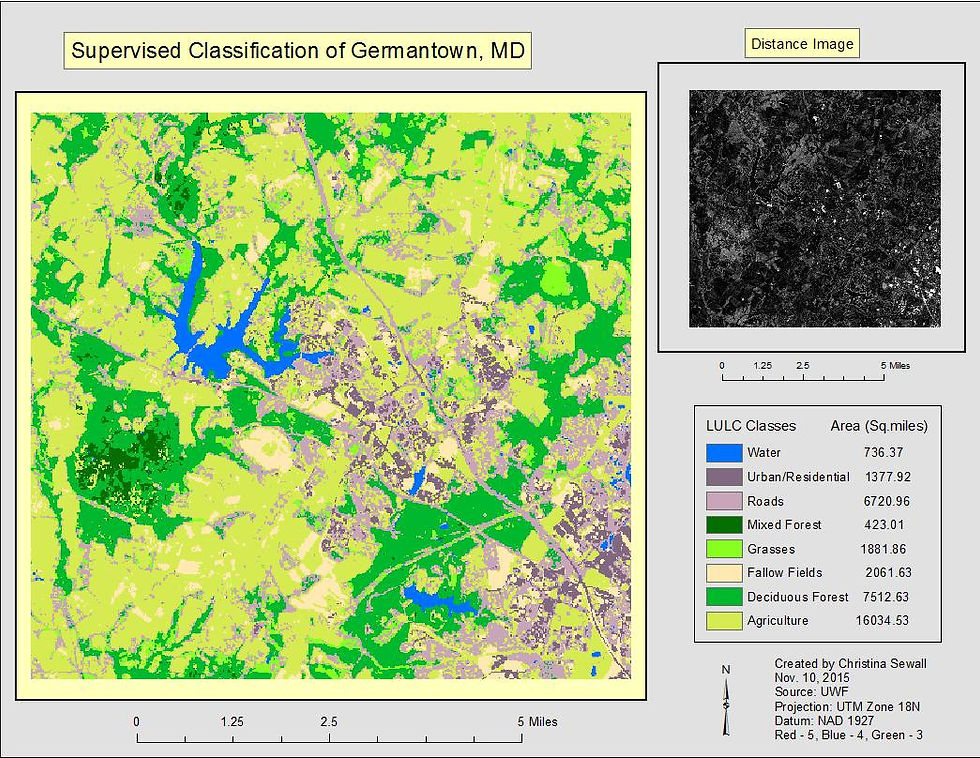
This map shows a supervised classification to identify land use and land cover in Germantown, MD for the purposes of tracking changes in population and land consumption. An image of the area was used to create signatures to be used in classification, and based on those signatures, the image was classified and the signatures merged into 8 classes. The area for each class was calculated so that, when compared with images from previous or later years, the changes can be calculated.